Health Care
How AI Can Repair the Damaged Scientific Trial Course of
The scientific trial course of is damaged for quite a lot of causes, starting from the problem of designing trial protocols to the price of working them. However one important (and solvable) subject is discovering, enrolling, and retaining the correct sufferers on the proper time limit, effectively. Fifty-five % of all interventional trials terminated every year are terminated on account of lack of affected person enrollment.
These statistics paint a sobering actuality. Doubtlessly life-saving medication typically by no means develop into accessible to sufferers who’ve exhausted their probabilities with standard-of-care therapies. Physicians and healthcare techniques lose assets, effort, and time spent on analysis that’s finally terminated. Pharmaceutical firms lose investments made in a drug, whereas foregoing any potential income that drug might have produced. This administrative inefficiency is a malignancy that may and should be cured.
A part of the problem of enrolling the correct sufferers rapidly is that trial enrollment standards are sometimes extremely advanced. For a single trial, a affected person may have to fulfill 30 to 40 separate standards. So, slightly than merely in search of sufferers with breast most cancers, a trial could also be in search of sufferers with triple-negative breast most cancers, a particular BRCA2 mutation, no metastases to the mind, however different varieties of metastases, a historical past of getting tried a particular kind of immunotherapy however not yet one more—and different extremely particular traits.
In some circumstances, these standards are so strict {that a} adequate variety of eligible sufferers might by no means exist. Extra generally, nevertheless, the problem is temporal; whereas a affected person could also be a match one week, their situation could also be quickly altering, making them ineligible for a similar trial just some weeks later. That is particularly widespread with most cancers, which is, by its nature, a progressive situation. If a affected person is just not recognized throughout the slender window of time throughout which they qualify for the trial, they won’t meet the standards for participation.
Underneath the present system, physicians working the analysis at a hospital (principal investigators), both await different docs to refer sufferers (which might take years–particularly for uncommon circumstances), or they’ve their staff of scientific analysis coordinators performing guide evaluation of affected person charts for matches, which will be cost-prohibitive at $100 an hour and as much as half-hour per chart (or digital medical document). Furthermore, by the point these scientific analysis coordinators have learn some recordsdata in week one, those self same recordsdata or remaining recordsdata might have modified by week two or three. It’s not humanly doable for a researcher to learn and course of the info quick sufficient to search out sufferers throughout their window of alternative.
Unleashing the potential of AI
One promising resolution is utilizing a sort of synthetic intelligence (AI) known as pure language processing to evaluation clinicians’ notes through digital well being data to search out sufferers eligible for scientific trial participation. It’s a course of that takes seconds. However there’s a primary step that’s important earlier than deploying the AI, which is streamlining the trial eligibility standards in order that the queries will be goal and deterministic find the precise information or related insights chronologically described within the doctor’s notes.
This means of streamlining begins with deconstructing current standards into discrete information queries or “questions.” If a trial sponsor is in search of sufferers with metastatic, castration-resistant prostate most cancers, for instance, there are greater than three information factors the AI will likely be in search of in affected person charts. One information level is metastasis standing. One other is a prognosis of prostate most cancers. Castration-resistance is a little more sophisticated. If a clinician has not used these phrases particularly of their notes, the AI will should be programmed to algorithmically “know” that rising PSA ranges whereas testosterone ranges are lowering signifies an indication of castration-resistant illness. Subsequently, it might want to then discover a number of information factors displaying the affected person’s PSA and testosterone ranges over time.
In essence, protocol scoping means turning a posh protocol into easy computer-friendly queries, just like taking the chemical composition of meals and turning it right into a recipe for a particular dish.
The method described requires subtle Pure Language Understanding (NLU) capabilities. Traditionally, NLU for scientific notes has been synonymous with Named Entity Recognition (NER), a shallow approach that merely identifies entities (e.g., medicines, ailments, diagnostic procedures) within the textual content of a scientific notice. Nonetheless, NER doesn’t present the form of structured relational semantics which are needed for a real understanding of scientific notes. It would determine a point out of a drug title akin to prednisone, but it surely won’t let you know what the scientific notice is saying about prednisone (e.g., whether or not it was began, stopped, resumed, or whether or not and why the dosage was elevated or decreased). True NLU capabilities are wanted to generate nuanced insights from in depth notes in medical data.
Rising consolation with AI amongst clinicians and the general public
AI remains to be a piece in progress, but it surely’s useful to recollect how rapidly the expertise is advancing. It was only some years in the past after we have been asking Siri the climate, and now, we’re utilizing AI to evaluation unstructured information in a clinician’s notes with close to human accuracy for a lot of scientific traits.
The final word aim is to enhance therapy choices for sufferers—and prolong lives. Take a situation like uveal melanoma with metastasis to the liver, a uncommon type of most cancers that has a mean life expectancy of 16 months after prognosis. Since there are not any FDA-approved therapy choices for the illness, the present customary of care is to refer eligible sufferers for scientific trials. As of March of this yr, solely 11 scientific trials had been initiated for this situation since 2011 within the US; three of these have been terminated, and in complete, fewer than 400 sufferers have been enrolled in all of the scientific trials. With out entry to a scientific trial, these sufferers solely have a 6% chance of survival. So it’s not an overstatement to say that by accelerating the recruitment course of and bettering the effectivity of scientific trials, AI can save lives.
Regardless of the promise of AI within the scientific trial enrollment course of, overcoming clinician resistance stays an impediment. There’s worry amongst some docs that AI seeks to switch them. However AI-driven instruments shouldn’t work together with sufferers, and even know their identities. Their aim needs to be merely to evaluation de-identified and uncooked medical information to search out sufferers who’re eligible for lively scientific trials. The dialog about therapy choices, trial participation choices, and different care plan selections ought to nonetheless at all times between the clinician and the affected person.
I’m hopeful that as clinicians and trial sponsors see what cutting-edge AI instruments can do, they’ll develop into extra snug with the capabilities of latest applied sciences. By combining AI with human experience and oversight, we have now the potential to revolutionize the scientific trial course of—and most significantly, prolong and save lives.
Picture: Deidre Blackman, Getty Photographs
Related Posts
- Heard at HLTH: Healthcare Execs Share Views on AI, Digital Well being and Worth-based Care
On the HLTH 2023 occasion earlier this month, executives from a number of the largest…
- Butterfly Community Launches AI Software for Level-Of-Care Lung Ultrasounds
In April, Butterfly Community acquired FDA clearance for its AI instrument designed to assist suppliers…
- What's scientific zero belief?
Safety has at all times been essential in healthcare, however with the speedy development of…
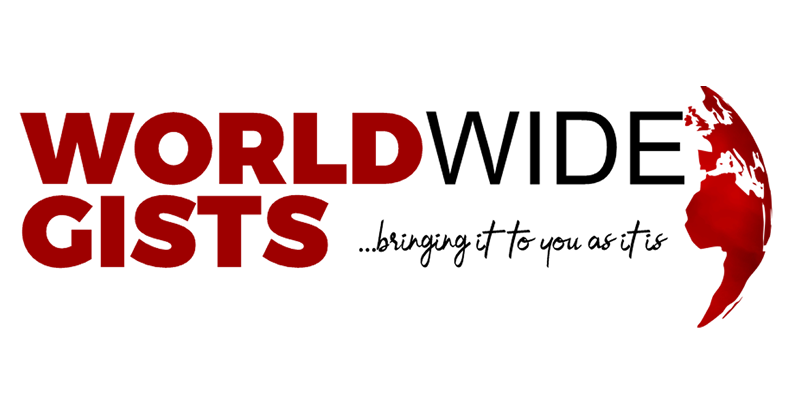