Health Care
The Chatbots Might Poison Themselves
To start with, the chatbots and their ilk ate up the human-made web. Numerous generative-AI fashions of the kind that energy ChatGPT acquired their begin by devouring information from websites together with Wikipedia, Getty, and Scribd. They consumed textual content, pictures, and different content material, studying by way of algorithmic digestion their flavors and texture, which components go properly collectively and which don’t, with a view to concoct their very own artwork and writing. However this feast solely whet their urge for food.
Generative AI is completely reliant on the sustenance it will get from the online: Computer systems mime intelligence by processing virtually unfathomable quantities of information and deriving patterns from them. ChatGPT can write a satisfactory high-school essay as a result of it has learn libraries’ value of digitized books and articles, whereas DALL-E 2 can produce Picasso-esque pictures as a result of it has analyzed one thing like all the trajectory of artwork historical past. The extra they prepare on, the smarter they seem.
Ultimately, these applications may have ingested virtually each human-made little bit of digital materials. And they’re already getting used to engorge the online with their very own machine-made content material, which is able to solely proceed to proliferate—throughout TikTok and Instagram, on the websites of media retailers and retailers, and even in educational experiments. To develop ever extra superior AI merchandise, Huge Tech might need no alternative however to feed its applications AI-generated content material, or simply won’t have the ability to sift human fodder from the artificial—a probably disastrous change in weight loss plan for each the fashions and the web, in response to researchers.
Learn: AI doomerism is a decoy
The issue with utilizing AI output to coach future AI is easy. Regardless of beautiful advances, chatbots and different generative instruments such because the image-making Midjourney and Steady Diffusion stay typically shockingly dysfunctional—their outputs full of biases, falsehoods, and absurdities. “These errors will migrate into” future iterations of the applications, Ilia Shumailov, a machine-learning researcher at Oxford College, advised me. “Should you think about this occurring again and again, you’ll amplify errors over time.” In a latest examine on this phenomenon, which has not been peer-reviewed, Shumailov and his co-authors describe the conclusion of these amplified errors as mannequin collapse: “a degenerative course of whereby, over time, fashions overlook,” virtually as in the event that they have been rising senile. (The authors initially known as the phenomenon “mannequin dementia,” however renamed it after receiving criticism for trivializing human dementia.)
Generative AI produces outputs that, based mostly on its coaching information, are most possible. (As an example, ChatGPT will predict that, in a greeting, doing? is prone to observe how are you.) Which means occasions that appear to be much less possible, whether or not due to flaws in an algorithm or a coaching pattern that doesn’t adequately mirror the true world—unconventional phrase selections, unusual shapes, pictures of individuals with darker pores and skin (melanin is commonly scant in picture datasets)—is not going to present up as a lot within the mannequin’s outputs, or will present up with deep flaws. Every successive AI educated on previous AI would lose info on unbelievable occasions and compound these errors, Aditi Raghunathan, a pc scientist at Carnegie Mellon College, advised me. You might be what you eat.
Recursive coaching may amplify bias and error, as earlier analysis additionally suggests—chatbots educated on the writings of a racist chatbot, comparable to early variations of ChatGPT that racially profiled Muslim males as “terrorists,” would solely develop into extra prejudiced. And if taken to an excessive, such recursion would additionally degrade an AI mannequin’s most elementary capabilities. As every technology of AI misunderstands or forgets underrepresented ideas, it would develop into overconfident about what it does know. Ultimately, what the machine deems “possible” will start to look incoherent to people, Nicolas Papernot, a pc scientist on the College of Toronto and one in every of Shumailov’s co-authors, advised me.
The examine examined how mannequin collapse would play out in varied AI applications—assume GPT-2 educated on the outputs of GPT-1, GPT-3 on the outputs of GPT-2, GPT-4 on the outputs of GPT-3, and so forth, till the nth technology. A mannequin that started off producing a grid of numbers displayed an array of blurry zeroes after 20 generations; a mannequin meant to type information into two teams ultimately misplaced the power to differentiate between them in any respect, producing a single dot after 2,000 generations. The examine supplies a “good, concrete approach of demonstrating what occurs” with such an information suggestions loop, Raghunathan, who was not concerned with the analysis, mentioned. The AIs wolfed up each other’s outputs, and in flip each other, a type of recursive cannibalism that left nothing of use or substance behind—these should not Shakespeare’s anthropophagi, or human-eaters, a lot as mechanophagi of Silicon Valley’s design.
The language mannequin they examined, too, utterly broke down. This system at first fluently completed a sentence about English Gothic structure, however after 9 generations of studying from AI-generated information, it responded to the identical immediate by spewing gibberish: “structure. Along with being residence to among the world’s largest populations of black @-@ tailed jackrabbits, white @-@ tailed jackrabbits, blue @-@ tailed jackrabbits, crimson @-@ tailed jackrabbits, yellow @-.” For a machine to create a purposeful map of a language and its meanings, it should plot each potential phrase, no matter how frequent it’s. “In language, you must mannequin the distribution of all potential phrases that will make up a sentence,” Papernot mentioned. “As a result of there’s a failure [to do so] over a number of generations of fashions, it converges to outputting nonsensical sequences.”
In different phrases, the applications may solely spit again out a meaningless common—like a cassette that, after being copied sufficient instances on a tape deck, feels like static. Because the science-fiction writer Ted Chiang has written, if ChatGPT is a condensed model of the web, akin to how a JPEG file compresses {a photograph}, then coaching future chatbots on ChatGPT’s output is “the digital equal of repeatedly making photocopies of photocopies within the outdated days. The picture high quality solely will get worse.”
The chance of eventual mannequin collapse doesn’t imply the expertise is nugatory or fated to poison itself. Alex Dimakis, a pc scientist on the College of Texas at Austin and a co-director of the Nationwide AI Institute for Foundations of Machine Studying, which is sponsored by the Nationwide Science Basis, pointed to privateness and copyright issues as potential causes to coach AI on artificial information. Contemplate medical purposes: Utilizing actual sufferers’ medical info to coach AI poses large privateness violations that utilizing consultant artificial information may bypass—say, by taking a group of individuals’s information and utilizing a pc program to generate a new dataset that, within the combination, accommodates the identical info. To take one other instance, restricted coaching materials is obtainable in uncommon languages, however a machine-learning program may produce permutations of what’s accessible to enhance the dataset.
Learn: ChatGPT is already out of date
The potential for AI-generated information to end in mannequin collapse, then, emphasizes the necessity to curate coaching datasets. “Filtering is an entire analysis space proper now,” Dimakis advised me. “And we see it has a huge effect on the standard of the fashions”—given sufficient information, a program educated on a smaller quantity of high-quality inputs can outperform a bloated one. Simply as artificial information aren’t inherently unhealthy, “human-generated information is just not a gold customary,” Ilia Shumailov mentioned. “We’d like information that represents the underlying distribution properly.” Human and machine outputs are simply as prone to be misaligned with actuality (many current discriminatory AI merchandise have been educated on human creations). Researchers may probably curate AI-generated information to alleviate bias and different issues, by coaching their fashions on extra consultant information. Utilizing AI to generate textual content or pictures that counterbalance prejudice in current datasets and pc applications, as an example, may present a solution to “probably debias techniques by utilizing this managed technology of information,” Aditi Raghunathan mentioned.
A mannequin that’s proven to have dramatically collapsed to the extent that Shumailov and Papernot documented would by no means be launched as a product, anyway. Of better concern is the compounding of smaller, hard-to-detect biases and misperceptions—particularly as machine-made content material turns into tougher, if not unattainable, to differentiate from human creations. “I feel the hazard is basically extra once you prepare on the artificial information and consequently have some flaws which can be so refined that our present analysis pipelines don’t seize them,” Raghunathan mentioned. Gender bias in a résumé-screening device, as an example, may in a subsequent technology of this system morph into extra insidious varieties. The chatbots won’t eat themselves a lot as leach undetectable traces of cybernetic lead that accumulate throughout the web with time, poisoning not simply their very own meals and water provide, however humanity’s.
Related Posts
- AI Order for Health Care May Bring Patients, Doctors Closer
You might have used ChatGPT-4 or one of many different new synthetic intelligence chatbots to…
- Self-Care With Endometriosis
SOURCES:Cara King, DO, director, benign gynecologic surgical procedure; affiliate program director of minimally invasive gynecologic…
- How Medicaid Directors Are Thinking About AI
During an Oct. 25 National Academy of Medicine Workshop on Generative AI and Large Language…
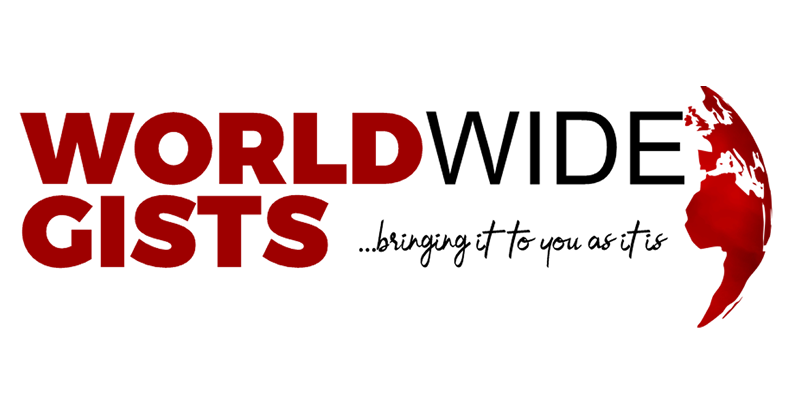